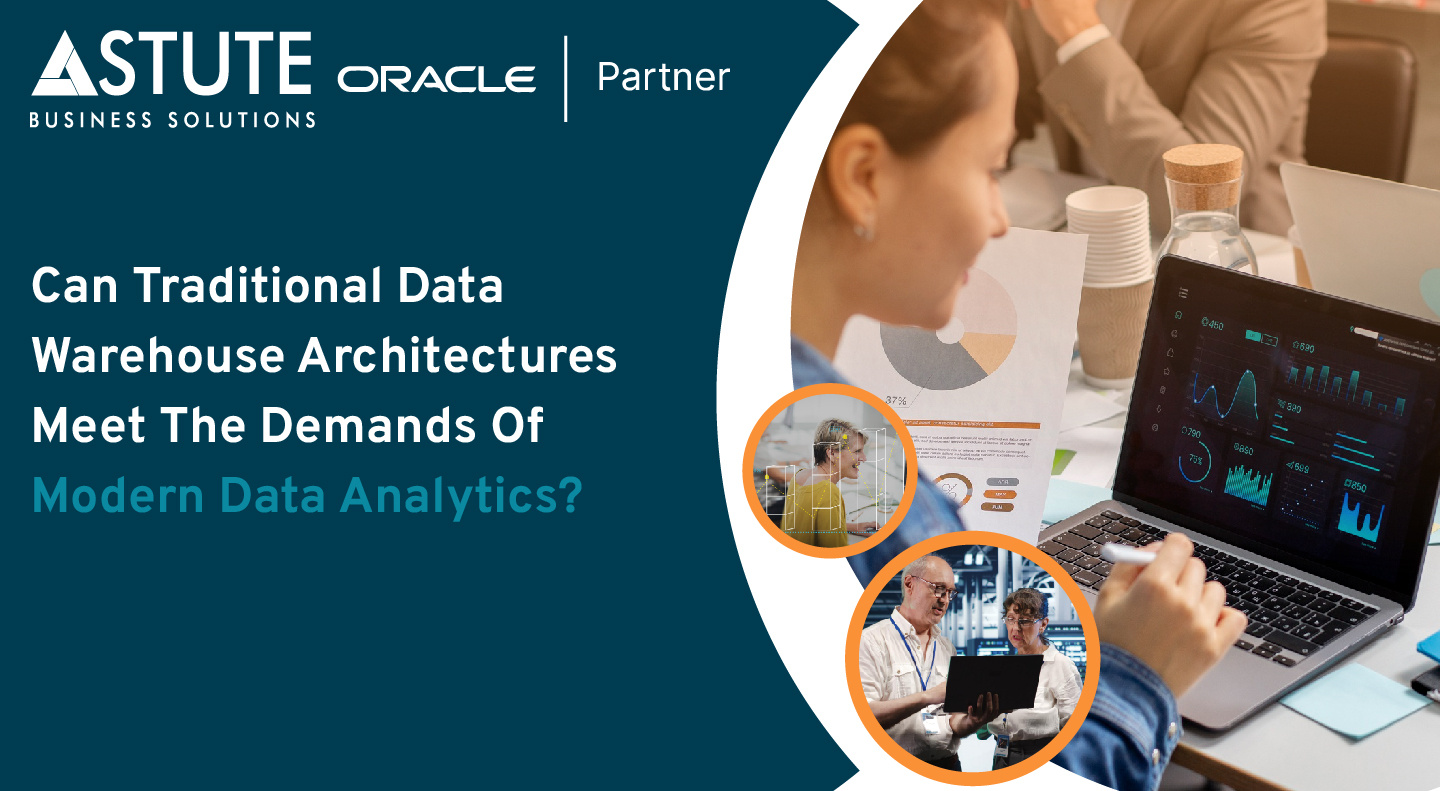
Can Traditional Data Warehouse Architectures Meet The Demands Of Modern Data Analytics
Table of Contents
In today’s fast-evolving digital landscape, businesses rely on data analytics for strategic decision-making, operational efficiency, and customer insights. However, the question arises: Can traditional data warehouse architectures still meet the growing demands of modern-day data analytics? With increasing data complexity, volume, and the need for real-time insights, organizations are re-evaluating their data strategies.
The Evolution of Data Warehousing
Traditional data warehouse architectures were designed primarily for structured data, with batch processing and rigid schemas. They excelled at historical reporting but struggled with agility, real-time data processing, and the ability to integrate diverse data sources. Today, enterprises need more than just a centralized repository; they require a modern data platform that supports automation, scalability, and AI-driven insights.
Table Stakes for a Modern Data Platform
Businesses need a modern data warehouse architecture that consists of the following to remain competitive:
Streamlined Data Migration and ETL Automation
Customers now expect data migration and ETL (Extract, Transform, Load) processes to be automated, simplified, and streamlined. Traditional ETL processes that require extensive manual intervention and lengthy development cycles are no longer sustainable. Modern data platforms leverage AI-driven ETL, real-time ingestion, and self-service data preparation to reduce complexity and accelerate time-to-insight.
Natural Language Processing (NLP) for Data Querying
The impact of AI on data analytics is profound. Users now expect to interact with data using natural language queries rather than SQL or predefined dashboards. AI-powered analytics platforms enable users to ask complex questions in plain English (or any language) and receive actionable insights in real time. Furthermore, visualization tools are expected to interpret NLP queries and present results in an intuitive, visually rich format—eliminating the need for specialized data expertise.
Data Quality and Data Integration
Poor data quality can undermine even the most advanced analytics initiatives. Organizations require data platforms with built-in capabilities for data cleansing, deduplication, and enrichment. Additionally, seamless integration with cloud applications, IoT data streams, and external data sources is essential to ensure a holistic view of business performance.
Data Security and Compliance
With data breaches and regulatory requirements on the rise, security is a top priority. A modern data warehouse must include encryption, role-based access control (RBAC), and compliance with global standards such as GDPR, CCPA, and HIPAA. AI-driven anomaly detection further strengthens security by identifying and mitigating threats in real time.
Top 5 Criteria for Selecting a Modern Data Platform
When evaluating a modern data platform for data warehousing, data lakes, and analytics, organizations should consider the following key criteria:
1. Scalability and Performance
The platform must handle growing data volumes, support real-time analytics, and provide high-performance query capabilities, ensuring that insights are delivered at the speed of business.
2. Flexibility and Multi-Cloud Support
Organizations need a platform that supports hybrid and multi-cloud environments, enabling them to deploy workloads seamlessly across different cloud providers and on-premises infrastructures.
3. AI and Automation Capabilities
AI-driven automation for ETL, data governance, and predictive analytics is crucial to reduce manual effort and enhance data-driven decision-making across the enterprise.
4. Comprehensive Data Governance and Security
A robust data platform should include built-in governance, lineage tracking, access controls, and compliance adherence to ensure data integrity, privacy, and security.
5. Ease of Use and Self-Service Analytics
The platform should empower business users with self-service analytics, allowing them to explore data, generate reports, and derive insights without relying on IT teams or data specialists.
Legacy Data Warehousing vs. Oracle Analytics Cloud with Autonomous Data Warehouse
Legacy data warehousing solutions often struggle with the following:
- Rigid, on-premises infrastructure that lacks scalability and agility.
- Manual ETL processes that require extensive time and effort.
- Limited AI/ML capabilities, making predictive analytics difficult.
- Fragmented data integration, leading to siloed insights.
- Complex security configurations that require constant monitoring and updates.
In contrast, Oracle Analytics Cloud with Autonomous Data Warehouse (ADW) provides:
- Natively built-in AI and ML capabilities, enabling advanced analytics without additional configuration.
- Automated data ingestion, transformation, and performance tuning, significantly reducing manual workload.
- Natural language-based querying and AI-driven insights allow business users to derive value effortlessly.
- Seamless data integration across multi-cloud and on-premise environments, ensuring a unified data view.
- Autonomous security and compliance enforcement, minimizing risks and ensuring regulatory adherence.
The Path Forward
Businesses that rely on traditional data warehouses without modernization efforts risk falling behind. The shift towards cloud-based, AI-driven data platforms is inevitable. Organizations must adopt architectures that enable:
- Real-time analytics rather than batch processing.
- AI and machine learning-driven insights rather than static reports.
- Self-service and NLP-based querying rather than IT-dependent report generation.
- Automated ETL and data integration rather than manually intensive workflows.
- Enhanced security and compliance are fundamental design principles.
By embracing a modern data warehouse strategy—such as Oracle Analytics Cloud with Autonomous Data Warehouse—organizations can unlock the full potential of their data, enhance decision-making, and drive competitive advantage. The future of data analytics belongs to agile, AI-powered, and user-friendly platforms, ensuring that every business user—not just data scientists—can derive meaningful insights effortlessly.
Ratnakar Nanavaty is the Chief Strategist of Astute Business Solutions. For the past 30 years, he has helped 80+ higher education, government, non-profit, public, and private institutions in various capacities. His expertise lies in transition leadership—bringing change to culture, strategy, management, and the revamping of Information Technology Departments. He specializes in assessing, mapping a change strategy, and institutionalizing change.
Search
Related Posts
Subscribe Our Newsletter
Gain access to exclusive insights, technical know-how and crucial knowledge from Astute experts.
Share Article
Reach Out